Introduction
Predictive analytics use a variety of techniques (e.g., statistical methods, machine learning algorithms) to make predictions about future events. 1 Although predictive analytics have been used in many disciplines since the early 20th century (e.g., actuarial science, business intelligence, financial forecasting), increasingly, this computationally intensive technique has been employed by law enforcement and other government agencies to generate predictions about future criminal behavior. 2
While the function of predictive analytics in policing appears to be well intentioned, upon a closer examination, one may find that the decisions generated by ‘black-box’ modeling techniques like supervised machine learning encourage a ‘blind faith’ in the mathematical process and in the ability of algorithms to produce meaningful and informative predictions. 3 Thus, despite their widespread use, predictive policing tools are complex systems with many aspects that remain a mystery.
In this paper, we critically examine the employment of predictive analytics in US criminal justice policy, with a particular focus on the ways in which these technological practices are reproducing and reinforcing structural relations of difference. We are specifically motivated by the following questions: In what ways do the algorithms become part of a larger sociotechnical apparatus of sociopolitical relations? How are the data that the algorithms inherit always-already imbued with structural relations of difference? To what extent do algorithms predict or performatively enact differential patterns of sentencing decisions or recidivism? In what ways might bodies, spaces, and practices be materially formed and shaped from the performative acts of algorithmic legal reasoning? And, in what ways might the cloaking of algorithmic legal reasoning as objective, precise, and efficient enable the proliferation of racializing assemblages in the juridical field? Each of these motivating questions will be examined through a new materialist lens that posits algorithms as more-than-human ontologies and racializing assemblages.
As argued by the first author elsewhere, we need to move beyond the dominant tendency to treat algorithms as mechanical operations that are contingent on human intervention or design and, as such, a prosthetic tool to human cognition. 4 The concept of algo-ritmo reconceptualizes the algorithm as engaging in more-than-human performative acts that are produced from the immanent forms of what Luciana Parisi refers to as the “soft” thought of algorithmic reasoning. 5 As a concept, algo-ritmo not only accounts theoretically for how the more-than-human performative acts of algorithms function but also considers the ways in which those algorithmic acts are forming, shaping, hierarchizing, and differentiating bodies. Thus, algo-ritmo also refers to the ways in which algorithms become racializing assemblages. As articulated by Alex Weheliye, racializing assemblages are a system of sociopolitical relations that hierarchizes and differentiates bodies, designating bodies as human, not-quite-human, and nonhuman; rendering certain bodies as exceptional and others as disposable. 6 Algo-ritmo postulates that the bodily disciplining is both human and more-than-human. Thus, there are not just human bodies that are racialized but algorithms too. In other words, we argue that inside of the proverbial “black box” 7, algorithms become immanent agencies of racializing assemblages. By doing a close reading of policy documents and existing research literature, we critically examine the racializing assemblages of algorithmic law enforcement logic, policies and practices, followed by ethical and social policy considerations. We begin by discussing the literature on big data and the rise of predictive policing.
Policing Crime & Predictive Analytics
Big Data & The Rise of Predictive Policing
In recent years, the private sector and government have focused their efforts on harnessing the power of big data to find meaningful patterns and make data-driven decisions. Private corporations such as Target and Walmart have analyzed their customer data to inform marketing strategies 8 and product sales and placement. 9 Government agencies are also leveraging big data and analytics to improve policy decision-making. Most notably, in 2012, the Obama Administration unveiled a Big Data Initiative, which sought to “improve the tools and techniques needed to access, organize, and glean discoveries from huge volumes of digital data.” 10 Arguably, where there have been even more advancements on these fronts has been in local city governance and institutional practices. While many government institutions have turned to big data analytics to make more “intelligent” decisions, law enforcement and criminal justice have emerged as two of the most prevalent areas in which these techniques have been developed and applied.
Policing Bodies Numerically
Before delving into the use of analytics in policing, it is important to understand the history of the use of statistics and numbers more broadly in criminal justice research. Prediction and forecasting have been used for nearly a century to study crime in the U.S. While much of this research has been focused on studying criminal behavior 11, other work has used forecasting methods to predict trends in crime and prison populations. 12 That said, no matter the outcome, criminal justice researchers have long been obsessed with using data—and in particular quantitative data—to understand crime. One prevailing belief is that, culturally speaking, the predominance of quantitative methods in criminal justice research reflects a broader societal notion about what it means to do science, and in this context it should not be surprising that to do science requires the manipulation and calculation of numbers. 13
The act of defining and explaining social phenomena using numbers or quantitative data is nothing new. In fact, this phenomenon has its roots in positivism. Positivism refers to the philosophical writings (circa 1830-1842) of Auguste Comte in which the scientific method—the standard procedure for gaining and producing knowledge in the natural sciences—was applied to the study of human behavior and social phenomena. 14 According to Comte, there are three premises of positivism:
- Universal truths exist for human behavior and social phenomena.
- Empirical observation is the only rational means by which universal truths in the social world can be discovered.
- Through the application of the scientific method, causal relationships among social phenomena can be established
Imbued within positivist philosophy is the notion that truth cannot only be discovered but also replicated through (precise and certain) scientific measurement. It was through the application of positivism to the study of the human condition and social life that quantification and the scientific method become central features of social and behavioral science and the preferred ways of understanding populations. 15
While the rise of positivism led to the growing use of quantitative methods in the human sciences, why were these methods so readily and widely accepted? Theodore Porter in his seminal work Trust in Numbers historically traces the rise of objective empiricism and ubiquity of quantification in the modern world. 16 To Porter, and largely society as a whole, we are drawn to numbers not only because they can be used to describe social phenomena, but also because a decision ‘made’ by numbers appears impartial and indisputable. 17 This notion of numbers as ‘truth’ is rooted in the belief that mathematics is the language and logic of the natural world. When applied to the study of human and social life, however, in what ways and to what extent do numbers become political and reflect the normative assumptions about what is counted, how it is counted, and why it is counted? This critical question is at the heart of Porter’s work, and through the use of historical case studies he demonstrates that numbers are never void of subjectivity or judgment. 18 Rather, numbers are “strategies of communication” that are used to legitimize one’s actions and “provide standards against which [to measure progress and compare information].” 19 In this way, numbers become “an agency for acting on people [and] exercising power over them.” 20
If we build off Porter’s claim about the nature of numbers and take seriously the proposition that numbers are capable of exerting power, then we must consider the possibility that numbers, which can describe the social world, can just as easily be used to control it. Michel Foucault takes up this subject in many of his works, and characterizes discipline as a mode of power that “separates, analyses, differentiates…measures…and hierarchizes in terms of value.” 21 Importantly, for Foucault, while discipline is “exercised on the bodies of individuals…the individual is not the primary datum on which discipline is exercised.” 22 Although this statement may seem paradoxical, it can be explained by examining Foucault’s two lecture series Security, Territory, and Population and Governmentality. 23 In both works, Foucault provides a historical account of the evolution of government, and describes how society’s transition from feudalism to capitalism led government to be concerned with the population within its territory in order to successfully govern the territory over which it has control. Driving this concern was the desire to organize, optimize, and regulate state affairs. This, of course, required the collection of data from individuals, while privileging quantified population trends. Viewed through a Foucauldian lens, then, methods of quantification such as statistics are a means through which the state controls, categorizes, and classifies its citizens.
The Rise of the Carceral State
If statistics and numbers have had historical significance in criminal justice research, then we must inquire about the role they have played in policing bodies in the U.S. In particular, we ask how have numbers shaped policing and legitimized mass incarceration?
We contend that the origins of mass incarceration in the U.S. can be traced to the Johnson era’s War on Poverty. When Lyndon B. Johnson assumed the presidency, he not only vowed to enforce civil rights, but he also declared war on poverty. 24 After a few years in office, however, what began as a commitment to nationwide anti-poverty programs quickly turned into something more sinister—namely, an agenda to target and punish urban, black communities across America. 25
In 1965, Daniel Moynihan, a politician and sociologist, released a report titled The Negro Family: The Case for National Action. 26 In the report, Moynihan used employment data to identify the causes of poverty and social-ill (e.g., crime, corruption) in the U.S. Often citing dire statistics to shock the reader (e.g. “Almost One-Fourth of Negro Families are Headed by Females”), Moynihan’s report largely painted the poverty problem in the U.S. as a “negro problem” rooted in the disintegration of the black nuclear family. Though President Johnson would come to distance himself from the blatantly racist report authored by Moynihan, by the end of his term in office, the damage had already been done. The carefully crafted communication strategy embedded within Moynihan’s report had legitimized the government’s desire to control the “negro problem,” and culminated in the Omnibus Crime Control and Safe Streets Act of 1968. 27
Crime & Carceral State
The Omnibus Crime Control and Safe Streets Act of 1968 signaled a profound shift in the government’s attitude and response to the root causes and correlates of crime. 28 The Act enabled the federal government to provide financial assistance to local law enforcement agencies for the procurement of police equipment and laid the foundation for massive federal investments in crime control and prevention strategies. More importantly, however, the Act expanded the federal government’s (e.g., Department of Justice) role in local law enforcement and criminal justice matters, with the goal of creating an interconnected, coordinated system for the provision of justice and crime prevention. 29 Ultimately, the Crime Control and Safe Streets Act represented a major step by the government to federalize crime 30 and codified the prioritization of law enforcement over welfare as a means to address social problems. Less than a decade after President Johnson signed the Omnibus Crime Control and Safe Streets Act into Law, and after decades of stability in the rates of incarceration, the U.S. began to experience substantial growth in its prison population, a trend that has yet to be reversed.
By the late 1960s, fear of rising urban crime and drug related activities, coupled with social and political unrest, fueled bilateral support for the United States’ punitive approach to law and order. 31 This support translated into harsher punishments for minor offenses and mandatory minimum sentences and, ultimately, resulted in extraordinarily high rates of incarceration after 1970. According to estimates published by the Bureau of Justice Statistics, approximately 500,000 people were incarcerated in 1980. By 2000, however, the total incarcerated population had exploded to more than 1.9 million. 32 Though the shift towards tougher penal policies exerted a significant influence on national incarceration rates, time trends show that growth in incarceration in the U.S. has not been uniform across offenses or offenders.
It is commonly recognized that the War on Drugs has contributed to the rise in incarceration rates over the last 40 years. Thus, it should come as no surprise that drug offenses over time have begun to comprise a disproportionate share of arrests and prison admissions. Figure 1 shows the time trends from 1980 to 2012 in the rate of arrests for select crimes. The most striking pattern revealed in the figure is the sharp increase in the rate of arrests for drug abuse violations compared to arrests for other criminal offenses. Specifically, over the 32 years for which we have data, arrests for drug related offenses rose from 255.65 per 100,000 persons in 1980 to just under 500 per 100,000 in 2012, peaking at 633.25 per 100,000 persons in 2006. An examination of incarceration rates over the same period shows a similar pattern, where in 1980 the incarceration rate for a drug related offense was 15 per 100,000 persons, but by 2000, that rate had increased over tenfold to more than 150 per 100,000. 33
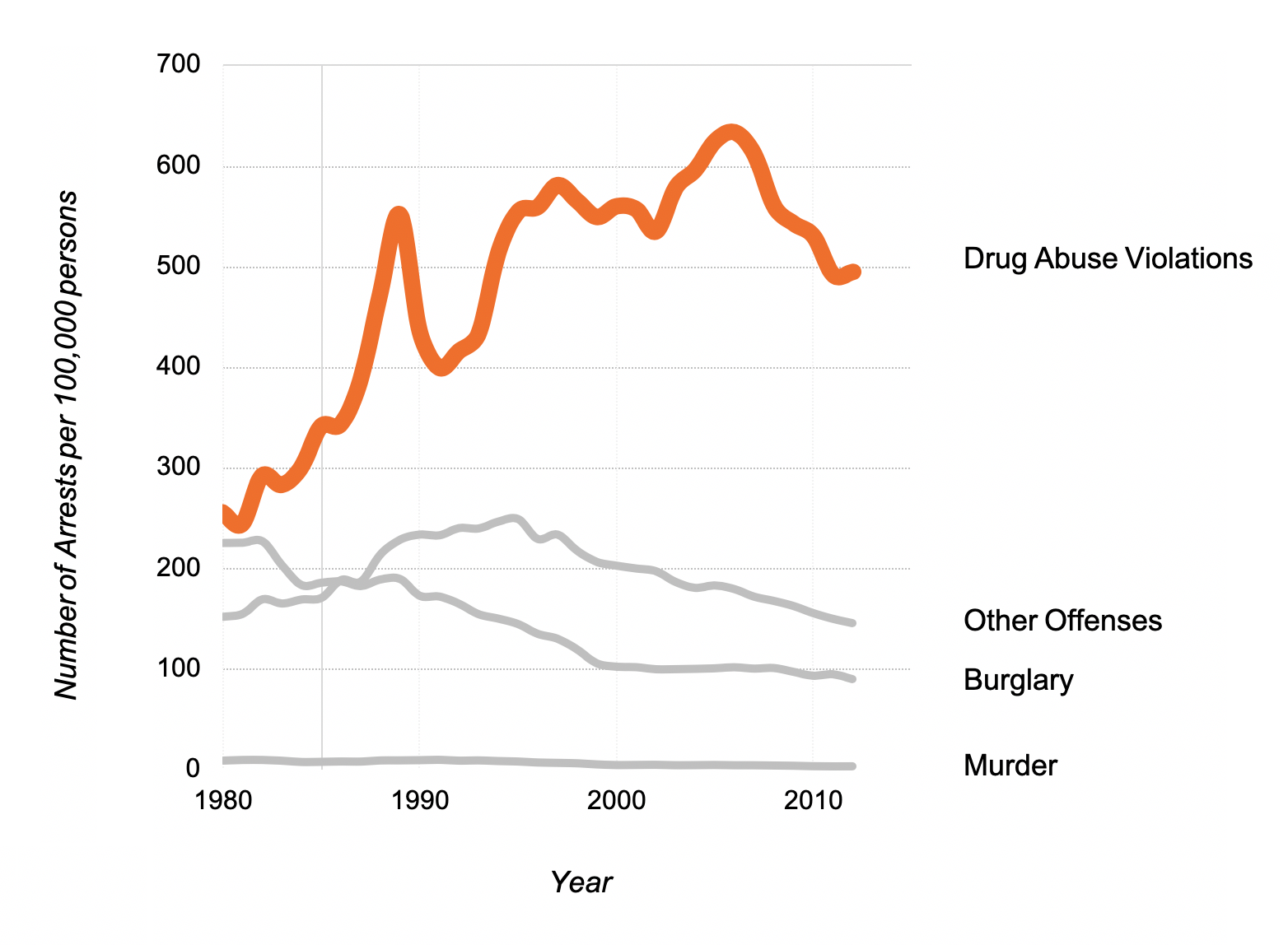
On the surface, the movement toward punitive crime control in the U.S. appeared to be broad and far-reaching. When disaggregated by race, however, the data present a more troubling picture. Despite comprising less than 12% of the U.S. population in 1980, African Americans made up 44% of the total amount of state and federal inmates in the same year. 34 By 2000, the population of African Americans in the U.S. had increased to 12.9%, and yet they accounted for 46% of all inmates in U.S. state and federal correctional facilities. 35 In the end, the U.S.’ policy of mass incarceration not only led to an overrepresentation of African Americans in jails and prisons, but it also reflects a clear policy choice to inscribe race into the criminal justice system. Perhaps John Ehrlichman, a former Nixon domestic policy chief, best expresses these sentiments:
“You want to know what this was really all about…The Nixon campaign in 1968, and the Nixon White House after that, had two enemies: the antiwar left and black people. You understand what I’m saying. We knew we couldn’t make it illegal to be either against the war or black, but by getting the public to associate the hippies with marijuana and blacks with heroin, and then criminalizing both heavily, we could disrupt those communities. We could arrest their leaders, raid their homes, break up their meetings, and vilify them night after night on the evening news. Did we know we were lying about the drugs? Of course we did.” 36
Policing in the Information Age
Today, across the U.S., dozens of police departments, from Boston to Los Angeles, have turned to sophisticated data analytics to address criminal justice practices. 37 Although the use of quantitative data to address crime is not novel, newer technologies fueled by algorithms, artificial intelligence, and machine learning enable law enforcement agencies to harness the power of big data to predict criminal behavior. This strategy is often referred to as predictive policing.
We adopt our definition of predictive policing from the RAND Corporation and National Institute of Justice. They define predictive policing as the “the application of analytical techniques—particularly quantitative techniques—to identify likely targets for police intervention and prevent crime or solve past crimes by making statistical predictions.” 38 Predictive policing and its tools permit law enforcement to use data to predict where crime is most likely to happen; assess who is most likely to (re)offend or be victimized; and form typologies of offenders. 39 While the goals of predictive policing may be clear, what remains muddied is what they are doing to accomplish these goals.
However, before we can examine what predictive policing tools are doing, we must have a better understanding of some of the keywords that are often used when discussing the subject, namely, artificial intelligence, supervised machine learning, and algorithm. Artificial intelligence is an umbrella term used to describe the science and engineering of creating machines and/or software with the ability to achieve a goal. 40, 41 Nested within AI is supervised machine learning. Supervised machine learning, as a branch of AI, is concerned with using historical information (i.e., a dataset that includes known input (x) and output (y) data) to train a model that can be used to predict future events (or behavior). 41 An algorithm, on the other hand, can be defined as a list of instructions for a step-by-step execution of a specific task. 43 Supervised machine learning algorithms, then, are computer programs that not only try to achieve a specific goal (e.g., predict the likelihood of crime in location Y) but also are capable of improving (or maintaining) their performance in service to that goal when fed new input data 41 As Foster and colleagues note:
“One key distinction in [supervised] machine learning is that the goal is not just to find the best function F that can predict Y for observed outcomes (known Ys) but to find one that best generalizes to new, unseen data.” 41
Machine learning algorithms have been applied to a variety of criminal justice contexts. These algorithms can be used at all stages of the criminal justice process, from determining where to deploy police officers for maximum impact 46, to informing bail decisions (also known as pretrial risk assessment) 47, to assessing risk for recidivating among parolees and probationers. 48 Police departments and other branches of the criminal justice system in cities around the country have adopted these tools, drawing on both researchers and university-based partnerships, as well as proprietary software, to develop and implement the desired algorithmic tools. One widely known proprietary tool, PredPol, is the product of a research partnership between UCLA, UC Santa Barbara, and the Los Angeles Police Department. According to their website, “PredPol’s mission is to provide a crime prevention platform to keep communities safer. Our…technology places public safety officers at the right time and location to give them the best chance of preventing crime.” 49 Using machine learning techniques, PredPol claims to be able to predict where crime is most likely to occur using only three data points: “crime type, crime location, and crime date and time.” 50 Another example is the COMPAS tool produced by Northpointe, which applies machine learning algorithms to risk assessment at the levels of policing and case management, promising users both a “prospective” and “retrospective look at risk and needs factors.” 51 Yet despite widespread use of these algorithms, touted as powerful and technologically advanced methods for improving public safety, their propensity to disproportionately target individuals from lower-class neighborhoods and communities of color remains starkly understudied and underreported outside of popular news media.
Algorithmic Reasoning as Racializing Assemblages
Machine learning algorithms are being employed in many sectors of US society including in the practices of predictive policing and criminal justice. Yet, we know less about the ways in which the algorithms may become part of a larger sociotechnical apparatus of sociopolitical relations. In order to examine this, we put the concept of algo-ritmo to work in analyzing the algorithmic legal reasoning of predictive policing. Algo-ritmo seeks to account for both the immanent agencies of algorithmic acts and the ways in which those acts become racializing assemblages.
In conceptually developing an understanding of how algorithms may become racializing assemblages, the first author has argued that it is through the data that the algorithms inherit sociopolitical relations of society. 52 Data are not pure, objective extractions of the world but rather are assemblages that are produced from a multiplicity of entwined and mutating apparatuses. The apparatuses of data assemblages include political economy, forms of knowledge, practices, governmentalities and legalities, and subjectivities and communities, among others. 53 As assemblages, they are both materially and discursively produced from forces of human and more-than-human ontologies. Among the multiplicity of forces that make up data assemblages include sociopolitical relations that consist of forces that differentiate and hierarchize bodies. Thus, all assemblages of data are always-already imbued with varying degrees of sociopolitical relations and, as such, become part of the (re)programmed architectures of algorithmic reasoning. 54
For algo-ritmo, algorithmic reasoning is not understood to be mechanical operations that are contingent on human intervention or design. It is postulated that the systematic operations of algorithms are not simply humanly designed and modeled or the prosthetic tool to human cognition. In accordance with Parisi, algorithms are understood to be actual entities that consist of finite operations of calculation as well as incomputable data sequences. As actual entities, they are sociotechnical ontologies that are always in process of becoming in relation with sociopolitical systems, legal practices, programmed inputs, and data assemblages. These are not simply humanly designed technologies, but rather as algorithms process and are trained on data assemblages they become more-than-human ontologies. For Parisi, it is the actuality of incomputabilities that provide instantiation of the immanent forms of algorithmic cognition. That is to say, operating between the space of finite algorithmic operations and the incomputability of the world’s infinite complexity (i.e., information) are forms of speculative reason that are immanent to computation. 55
If machine learning algorithms consist of immanent forms of reasoning, then the iterability of predictions or speech acts are more-than-human performative acts. Performative acts, or intra-actions, are relational acts within entangled entities that produce ongoing material (re)configurings of the world. 56 Building on Barad’s diffractive reading of Foucault’s discursive theory and Butler’s theory of performativity via Bohr’s conception of matter, algo-ritmo engages in more-than-human performative acts, in which materiality is discursive and the discursive is always-already material. It is via the iterable intra-actions with other relational ontologies that materially reconfigures the world. Thus, algo-ritmos matter through their iterable intra-actions with other ontologies, whereby they enact, form, shape, and produce both human and more-than-human bodies.
As agencies that inherit sociopolitical relations via data assemblages, the immanent forms of reasoning and more-than-human performative acts of algorithms become racializing assemblages. The theory of racializing assemblages seeks to more adequately account for the processes of power and racializations of the body/flesh. As argued by Weheliye, racialization is not to be reduced to race or racism but is the process of differentiation and hierarchization that produces the assemblages of race, gender, class, sexuality, and dis/ability among other structural relations of “difference”. 57 The sociopolitical relations of racialization are perpetuated via technologies and sciences (among other things) and require “the barring of nonwhite subjects from the category of the human.” 58 Thus, the data and code of algorithms inherit the sociopolitical relations of “difference” becoming a performative force of the relational and connected forces of racializing assemblages.
As a way of drawing a distinction between the legal constitution of the body and the social designations of the flesh, Weheliye also calls upon Hortense Spillers’ (2003) theorizing of the flesh. As Spillers insightfully states “before the ‘body’ there is ‘flesh,’ that zero degree of social conceptualization that does not escape concealment under the brush of discourse or the reflexes of iconography…” 59 Prior to the legal constitution of the body is the formation of the flesh, a formation that is bound by the markings or traces of political violence designating a hierarchy of humanity. The traces of political violence of the flesh are what Spillers refers to as “hieroglyphics of the flesh” that are produced from the instruments or acts of violence such as whips, police brutality, mass shootings, or more subtly from the silence in speech acts. The data and code of algorithmic acts inherit the sociopolitical forces and “hieroglyphics of the flesh” of racializing assemblages from the iterability of algorithmic intra-actions.
In this article, we examine the ways in which algorithmic legal reasoning, as materialized in practices of predictive policing, becomes racializing assemblages. As will be discussed below, sociopolitical relations of racializations become part of the algorithmic architecture in at least two ways. First is by way of the rationalities behind the metrics that operationalize and constitute the criterion of prediction. The second is more posthumanist via the algorithmic intra-action with data assemblages and through this process (re)configuring the architecture of the algorithm to becoming racializing assemblages. By putting the concept algo-ritmo to work in a close reading of the existing research and policy literature, we illuminate these sociopolitical processes.
Close Readings of Criminal Justic Research & Policy Literature
In this section, we present a case study of one application of predictive analytics in the City of Philadelphia criminal justice system, namely, the use of supervised machine learning algorithms to predict individuals’ risk of recidivating for the purpose of informing probation and parole decisions. We draw on a close reading of four key texts, which we have selected based on not only their exposition of the particular case but also their perceived importance in the wider body of criminal justice literature on this subject and their influence on informing criminal justice policy. These texts include a grant report 60, which provides an in-depth look at the long-term project of developing and implementing a machine learning-based risk assessment tool and its components, and three peer-reviewed journal articles, which report on various findings from the larger project. One of these journal articles 61 reports the results of a randomized controlled trial that resulted directly from the implementation of the machine learning algorithm, while the other two 62 focus on the algorithm itself. Each of these texts provides explicit instantiations of the rationalities that informed the development, use, and implementation of these algorithms in criminal justice policy and practice in the City of Philadelphia. Although we did not have access to the algorithms themselves, our close reading of these texts allows us to comment on the ways in which these algorithms may form and shape bodies, spaces, and practices through their application in this case example.
By engaging in close readings of these texts, our challenge is not to demonstrate that algorithms engage in racialized predictions. Findings from a recent study of racial bias in recidivism algorithms published in ProPublica 63 have already provided evidence for such patterns, demonstrating that one proprietary product employed in Broward County, Florida, falsely classified African American offenders as at high risk for recidivating nearly twice as often as it did white offenders. Instead, we seek to closely examine how the logic and the process behind the development, validation, and implementation of algorithms produce racialized, classed, and gendered predictions. In other words, we closely examine the ways in which the algorithms become racializing assemblages. We begin with a rejection of the notion of raw, pure data. As we discussed above, data are assemblages produced from multiple sociotechnical, sociocultural, and political forces, and are always-already imbued with structural relations of difference, in varying degrees. Far from providing us with a more “objective” means of classifying offenders, these algorithms inevitably inherit, reproduce, and potentially exponentiate human sociopolitical relations.
To a large extent, this process is guided by inputs. Inputs invariably reflect choices made by decision makers, data analysts, researchers, and algorithms, from decisions about data collection and context, to what predictors are included and excluded (and how they are measured). Likewise, these decisions include determinations about model parameters, encompassing not only the desired ratio of false negatives to false positives but also the operationalized definitions of risk categories. These choices include the symbolic representations and cultural understandings imbued in the data, in the algorithmic re-estimation of itself given intra-action with data, and in the values and rationales of the decision makers themselves. Thus, our reading of the texts focuses on an exploration of these various inputs and the ways in which they inform the ontological development of the algorithm.
It should also be noted that we have chosen to refer directly to the specific city and stakeholders involved in this case by name, rather than to employ the use of pseudonyms or vague language. In adopting a close reading methodology, our work requires us to include specific lines and passages from these texts, which would not be possible without detailed citations. We encourage readers to use these citations to conduct their own readings of the texts. Finally, given the widespread debate surrounding the problematic use of race in predictive analytics in criminal justice contexts, and its simultaneous centrality and inadequate treatment in the present case, we believe our decision to name the specific city to be ethical and in line with our desire not only to provide critique but also to encourage dialogue among all stakeholders involved.
Case Overview
The case explores the use of supervised machine learning algorithms as tools for informing decisions about the level and type of supervision required for adult probationers and parolees in Philadelphia. The Adult Probation and Parole Department (APPD) of Philadelphia began a research partnership with the University of Pennsylvania in the mid-2000s, the primary purpose of which “was to decide how best to use the Department’s scarce resources to prevent murder.” 64 This initial effort has yielded an ongoing collaboration around the development and implementation of “a criminal risk forecasting system…used since 2009 to assess all incoming probation and parole cases.” 65
Although we do not have access to the APPD’s criminal risk forecasting tool, it is reported by Barnes and Hyatt that a supervised random forest algorithm powers the system. Before discussing random forests, we must first define decision trees. Simply put, decision trees are a supervised learning method that develops a set of rules (e.g., if-then statements) that can be used to predict or classify some outcome of interest 66. Though many algorithms can build decision trees, APPD’s system grows trees through a technique called classification and regression trees (CART). 67 CART is an analytic method that recursively partitions a dataset into subgroups that are successively more and more homogeneous with respect to the outcome. 68 The specific partitioning procedure used depends on the distribution of the outcome; that is, a regression tree is developed when the outcome is continuous, while a classification tree is developed when the outcome is categorical. When visualized, the splits generated from the recursive partitioning form a tree-like structure. A random forest, then, can be thought of as a collection of decision trees. However, the random forest algorithm randomly selects inputs (i.e., predictors) and observations to build decision trees, and then makes predictions (or classifications) for each observation in the dataset by averaging the results of a collection of independent trees “into a single resultant outcome.” 69 According to its creators, “The resultant Risk Forecasting Tool is now used to assess all new probation cases [in the First Judicial District of Pennsylvania] at their outset, and allows the department to tailor its supervision protocols in a manner that reflects the danger that the individual probationers pose to the community.”70 Thus, the tool’s target population is adult probationers in the City of Philadelphia, although data for both probationers and parolees (i.e., adults “under supervision”71 by the First Judicial District) were used in developing and studying these tools and the inclusion of certain predictors. 72 The suggested goals of the project, gleaned from these texts, are to improve resource allocation, inform decision-making with regard to the level of supervision, and promote community safety and security. Although we acknowledge that this case is limited by its narrow scope, we believe that the themes illuminated by this case and these key texts illustrate and inform understanding of the process by which algorithms become racializing assemblages in the broader context of criminal justice. We turn now to our close reading and examination of the texts.
The Problematic of the Sociology of Race
The issue of using race in predictive analytics broadly permeates the criminal justice literature on this subject. The majority of this discussion focuses around the implications of using race as a predictor in machine learning algorithms for predicting recidivism, from the perspective of ethics, equity, and legality. The texts selected for the present case all take up, to varying extents and with varying aims, the use of race in prediction and decision making at the level of probation and parole. For instance, Berk explicitly focuses on the role of race as a predictor (input) in machine learning algorithms, demonstrating its predictive power relative to other variables in a model constructed using data on (predominantly) African American probationers and parolees.73 By contrast, Barnes and colleagues restrict any mention of race to their reporting of the demographic characteristics of the study sample.74 Although they mention that the sample of cases designated as “low-risk” by the machine learning algorithm and therefore eligible for inclusion in the RCT was “more likely to be white [and] less likely to be African-American”75 than the overall APPD caseload, they do not interrogate this distribution further, nor provide further comment on its potential impact on study outcomes. 76
In general, in these and other texts related to the application of machine learning approaches to risk assessment in criminal justice, the discourse surrounding race is confined to tests of its usefulness as a predictor in these models (i.e., its contribution to the overall predictive accuracy of the algorithms). The political and ethical dimensions of using race in this way are frequently mentioned, but the commentary is usually confined to concluding remarks. For instance, Barnes and Hyatt write in their conclusion, “Would it ever be permissible…to include an offender’s racial background as a predictor variable in one of these models? If not, what about the use of other predictors, such as residential location or familial circumstances, which could indirectly communicate the offender’s racial identity into the forecasting model?” 77
Furthermore, these musings often lead the authors to suggest that decisions about when to include or exclude race as a predictor in these algorithms should be made collectively in some fashion by stakeholders. Consider the following examples: “The democratic virtue of a statistical forecast is that it is transparent and debatable.”78 Further, “If the citizens of Philadelphia or their leaders prefer to take a small increase in forecasting error to gain a large improvement in the legitimacy of the entire process, that is exactly what an informed democracy can do.”79 Yet, despite these allusions to an implicitly democratic decision-making process regarding the design and implementation of these algorithms, the community members themselves—those who stand to be most affected by these tools—remain glaringly absent from the conversation. Other “stakeholders,” however, have indeed been taken into account, as evidenced by the following statement: “Nevertheless, the APPD leadership felt that the inclusion of certain predictors was politically necessary in order to defend the use of the model to various stakeholders associated with the city’s criminal justice system.” 80
Thus, three primary problems emerge, layering upon one another. First, when the authors do take up race, they treat it statistically rather than theoretically. Berk engages with race statistically through a discussion of how its shared covariance with other variables in the data renders it difficult—if not impossible—to remove race completely from the predictions of the algorithm. He writes, “When race is excluded from the set of predictors to begin with, the regions that race shares with gender and prior record are folded into gender and prior record,” adding further that “excluding race may then not matter much.” 81 This language can be interpreted as an algorithmic form of color-blind racism 82, whereby the racial inequality in the prediction of risk is assumed to be based on natural tendencies in human behavior and nonracial dynamics of inputs. The algorithm is used to justify racist institutional practices and decision making.
Second, in failing to take up any theoretical framings of race, the researchers either reject or ignore the recognition of race as a social construct. This neglect becomes problematic in their interpretation of the data, which are depicted as describing something inherent about the individual cases, rather than as revealing something about the forces of sociopolitical relations in a racialized society. 83 Such an interpretation allows the researchers to classify race and its proxies, such as zip codes, as inherent essentialist characteristics of the individual, rather than as byproducts of a system of racializations. Under this framing of race, researchers and decision makers can not only predict future behaviors of individuals (in which the risk score itself becomes another constructed attribute of the individual) but also speak with authority about what should be done to avoid them.
Third, through the use of race and proxies for race as predictors in machine learning algorithms, predictive analytics become a contemporary means of legitimating the racist and punitive social policies that date back to the Nixon era. With no grounding in or understanding of theories of the sociology of race, the algorithms become yet another byproduct of racializations in society, cloaking the work of the “new eugenics” beneath the auspices of a narrative of “objectivity.” The absence of community voices in the process of developing and implementing these algorithmic tools further amplifies the colonialist posturing exhibited in these texts.
Discourse on Risk and Cost
As previously described, the purpose of using machine learning algorithms at the probationary stage of criminal justice proceedings is to classify individuals according to an assessed level of risk of recidivating. In the APPD case, three levels were designated: low risk, moderate risk, and high risk. Individuals were classified as low risk if they were “not predicted to commit any new offenses, of any kind.” Moderate risk individuals were “predicted to commit only non-serious offenses,” while high risk individuals were “predicted to commit at least one serious offense – defined as murder, attempted murder, aggravated assault, robbery, or a sexual crime.” These categories encompassed the individuals’ predicted behaviors within two years of beginning a period of supervision under the APPD. 84
The meaning of risk in this context is thereby discursively formed according to the preferences of the decision makers at APPD and trained in the algorithm through statistical procedures designed by the model creators. Based on a logic of classification, individuals under surveillance are sorted into one of three categories, each with its own defined boundaries. This figurative ordering of bodies translates in the real world as an attempt to regulate and repress future deviance through adjusted or tailored levels of surveillance and participation in prescribed programming. Thus, these statistical procedures produce and reproduce cultural and social constructions of risk, obscured by a narrative of safety and security purported to reflect the views of certain communities, who, as noted above, have had no documented, direct involvement in the development of these tools. A stark example of this appears in Berk and colleagues, when they write, “African-Americans in Philadelphia have two major concerns about young black men in their community. One is the extraordinarily high levels at which they are incarcerated…the other is the very high rate at which they are murdered.” 85
Another critical dimension of supervised machine learning approaches is the degree to which cost language is embedded within it. “Cost” in this context is used not only to describe material and financial resources expended by the APPD in order to surveil and police bodies but also to denote decisions made in constructing the models themselves. Although error—defined as either a false negative or a false positive–is accepted as an “inevitable” 86 part of prediction in machine learning approaches, decision makers can decide which type of error is more “costly” and build this preference into the model as a cost ratio of false negatives to false positives among predicted high-risk cases. Because of the APPD’s “strong preference for erring on the side of caution when it came to making High Risk forecasts, the model is expressly designed to generate more High Risk false positives than false negatives.”87 Ibid., 46.] In other words, incorrectly identifying an individual as high-risk, and making decisions regarding the nature of that individual’s probation and parole accordingly, is considered less costly than failing to identify someone who goes on to commit a “serious offense” as defined above.
As described in these texts, machine learning approaches like random forest are not intended to test hypotheses and need not even be based in any theoretical justification. 88 Yet, despite the “black box” 89 nature of these algorithms, it is clear that inputs regarding levels of risk and cost ratios directly and deliberately influence model outputs. Moreover, these texts suggest that in the case of the APPD Risk Forecasting Tool, as well as the subsequent RCT to examine the outcomes associated with low-risk supervision programs, these decisions about model inputs reflect cost concerns of the decision makers. Consider the following passage: “[W]e cannot say that reduced supervision for low-risk probationers ‘works’ to reduce recidivism. What it does ‘work’ to do is cut costs for low-risk probationers, freeing up resources for higher-risk cases.”90 The discourse surrounding this tool attempts to portray it as beneficial to both the community, which enjoys improved safety and security, and the “high-risk offender,” who is able to receive individualized attention as a result of more tailored approaches to supervision.91
Construed as an opportunity to better inform decisions about how to allocate scarce resources, the tool actually serves as justification for greater supervision of individuals defined as “high risk” by the algorithm. Likewise, commentary around community “concerns” 92 falls flat when we recall that the communities themselves did not participate in the design, implementation, or testing of this tool. With a colonialist posture, the narrative of safety and security is thereby imposed on the areas most affected by this tool from the outside, and from the top down. In the APPD case, these regions are identified as Northeast and West Philadelphia, which are used as both the source of much of the data used to construct and validate the algorithms and the testing grounds for a new low-risk supervision approach informed by the risk assessment tool. No rationale is provided for the decision to focus the study on these particular neighborhoods, nor are regional demographic data reported by the authors. 93
Census data indicate, however, that neighborhoods in these regions tend to be characterized by lower-than-average median household incomes and higher proportions of nonwhite individuals. Citywide, Philadelphia has a median household income of $61,900, with 63.4 percent of the population reported as nonwhite. To put this in perspective, only one neighborhood in these two regions combined (Byberry, located in the northeastern-most corner of Northeast Philadelphia) has a median household income that either meets or exceeds the citywide median household income. Additionally, West Philadelphia’s neighborhoods, as well as the neighborhoods in southern Northeast Philadelphia, are characterized by high proportions of nonwhite citizens; the lowest proportion of nonwhite residents in West Philadelphia occurs in the Cobbs Creek neighborhood, in which 28.4 percent of the population reported white race/ethnicity on the Census. 94 Thus, the risk assessment tool is tested on a homogenous sample that is not representative of the City of Philadelphia population in terms of either race or class.
Further, the discourse around this tool mimics the language of a cost-benefit analysis, in which the costs are confined to mathematical ratios and supervisory resources, and the stakeholders are limited to department decision makers and their university-based collaborators. When the very definition of “risk” and one’s propensity to be classified as low, medium, or high risk is dependent on racialized, classed, aged, and gendered assumptions adopted and adapted by algorithmic reasoning, then the tool is not improving upon human inconsistencies but rather reproducing them in the form of a new datum – a risk score.
Data Quality
On a very fundamental level, the source and quality of the data used to create, validate, and refine risk assessment tools become a central concern when we consider that the processes by which algorithms become actual entities of racializing assemblages are initiated, and reiterated, through data inputs. In line with the cost considerations outlined above, researchers tasked with creating the risk assessment tool report, “All of the predictors used within these models were required to stem from data sources that were readily accessible…within the APPD’s data network.” 95 As such, all data used in the construction of these models were derived from administrative data made available and maintained by APPD. The authors do not describe the processes by which these data were originally collected, nor do they indicate whether the data undergo any kind of audit.
In terms of assessing model accuracy, the authors note the importance of using new data to validate the predictive accuracy of the model. For example, in Barnes and Hyatt, the “construction sample” and the “validation sample” are derived from probation case data from two different time periods (2002-2007 and 2001, respectively). 96 They argue that utilization of separate construction and validation samples is both “a ‘cleaner’ way to assess the model” and a means of extending the time horizon of analysis. 97 However, because both data samples are derived from the same APPD administrative database, the use of different samples for validation purposes does nothing to correct for potential inaccuracies in the data.
In the ProPublica analysis of a recidivism prediction tool, Angwin and colleagues note, “Defendants rarely have an opportunity to challenge their [risk] assessments. The results are usually shared with the defendant’s attorney, but the calculations that transformed the underlying data into a score are rarely revealed.” 98 The present texts do not discuss whether individuals under supervision by the APPD have the opportunity to verify their information prior to it being entered into the tool and used to produce a risk assessment score that will have long-term, material effects on their lives. Given that individuals do not have the ability to consent to a particular type of treatment with regard to the level of surveillance and/or associated services received 99, however, we suspect that their awareness of and opportunities to engage with these tools in this manner are minimal, suggesting that errors are likely to recur over time. The possibility, then, that these tools are constructed, validated, and implemented on the basis of inaccurate data cannot be ignored.
Moreover, the processes by which the data used in these algorithms are collected at various stages of criminal justice proceedings are not explored. For instance, in Berk 100, data used to analyze the contribution of race as a predictor to model accuracy among high-risk offenders encompassed both probationers and parolees under supervision by APPD. Although these matters are not discussed in the text, it seems likely that data collection procedures, times, and settings would vary for parolees and probationers, leading to uncertainty about the consistency and precision of data collection procedures for all individuals potentially coming into contact with risk assessment tools.
Notably, the specific predictors included and excluded in various versions of the model are outlined explicitly in the Barnes and Hyatt report. Unfortunately, this transparency does nothing to address overarching questions of data accuracy and quality, and uncertainty remains with regard to how these variables are measured. For instance, Barnes and Hyatt report using “the offender’s home zip code” as the basis for constructing several predictors, but they do not explain whether this zip code pertains to the offender’s last known permanent address, or some other measure.101 By contrast, the zip code included in the analysis by Berk is defined as “the zip code area into which an individual would be released.” 102 Given the dual role of zip code as both a measure of geography and a proxy for race and class, the precise definition of such a predictor becomes critical in the construction of these tools.
Furthermore, while researchers may develop and validate multiple versions of these models, using different predictors and/or data sets at each stage 103, the iterative nature of this process extends beyond the statistical or technical aspects of the algorithms to include also the ontologies of the decision makers themselves. At each step of model development, testing, and implementation, both algorithms and humans intra-act to advance their knowledge, using the results of the steps preceding to inform future decisions. As a result, it is not possible to restart this process completely from scratch, even if researchers were to use an entirely new dataset. While the machine may be wiped clean, the human remains a constant and deeply embedded part of this process, emerging in both predictable and unpredictable ways that make it difficult to detect or, perhaps, control. The recurrence of citations within the literature surveyed for the present case provides one example of this. On the one hand, the impact of past studies on future work illustrates the cumulative and reproducible nature of research, a familiar feature of the scientific process. On the other hand, it provides observable evidence of one of the ways in which the human experience becomes an integral and influential part of the process of conceptualizing, developing, and deploying machine learning tools. That is to say, the histor(icit)y of sociopolitical relations is an autopoietic sociotechnical system that consists of feedback loops both beyond and with the algorithm. It is precisely through this intra-play of machine and human intra-actions that these tools become imbued with structural relations of difference.
Discussion & Alternative Possibilities
Many social science and policy employments of big data analytics have maintained positivist orientations that assume that the algorithms are producing objective information about the world. This is especially the case with the use of supervised machine learning in predictive policing. In fact, as we point to in our close reading of the research and policy literature, there are at least two areas of inputs that influence the architectural formation of the algorithm. The first is by way of human discursive formations and, as such, operationalizations of “risk” and “cost,” and the second is by way of the algorithmic intra-actions with the data assemblages of administrative data. Thus, algorithms start with human reasoning, which informs their target and structure, but then become actual entities and immanent agencies as they intra-act with and ‘learn’ from the sociopolitical forces imbued in the data. We demonstrate through our close readings and argue that it is through both forms of input that algorithms become racializing assemblages. In other words, the algorithms become part of the assemblage of sociopolitical relations that hierarchizes and differentiates bodies in society.
If there are racial, class, and gendered disparities in both predictions of risk and false positive predictions of high risk (which were built into the algorithm) then there is also a legal question of disparate impact. Disparate impact refers to a policy that is found to have a disproportionate “adverse impact” against any group based on race, national origin, color, religion, or sex, among others, when there is no legitimate, non-discriminatory need for the policy. In this instance, the policy that produces disparate impact is inscripted into the architecture of the algorithm while masquerading as an objective instrument of predictions.
Alternative Possibilities
Given the way algorithms are designed and what they are doing and telling us, how might algorithms be reconsidered? What might be an alternative or re-imagined use of them? If we take seriously the subjective process of the performative acts of prediction, how might that shift how we approach the algorithm and its use? Again, given that data assemblages are always-already imbued with sociopolitical relations, culture, political economy, etc., how do we work with these complicated relational ontologies? We do have to wonder about the unreasonable effectiveness of the algorithms themselves, and how the algorithms do function with such “precision.” We unpack this by conceptualizing algorithmic prediction as a more-than-human performative force that intra-acts with data assemblages. But with this understanding, what might be the alternative possibilities? We now turn to discuss several implications from this work and consider their benefits and shortcomings.
As we have discussed, the construction of these algorithms for risk assessments was informed by a colonialist posture of what communities need and desire without including community voices. We argue that the lack of community voices has led to a criterion of pathology (i.e., risk) and performatively formed and shaped the individuals and the approaches to supporting their re-entry. Predicting the risk of committing a violent crime (i.e., pathology) performatively frames the material and discursive possibilities of approaches. Predicting risk is a deficit-based framing masquerading as an asset-based perspective, which legitimates power structures by characterizing individuals as unreliable, inherently “deviant” or “abnormal,” unable to speak for themselves, and dependent on the decisions, knowledge, and opinions of others. If the criterion were how well one can navigate institutions in society, then institutions would be better able to deploy the necessary resources to generatively enable human capacities, rather than constrain them. Even if the same predictors were found to be driving some of the prediction, such a shift reconfigures the material and discursive framing, use, and function of the algorithmic predictions and the possible approaches to supporting re-entry. Predictors do not capture something inherent about the subject but rather something inherent about the sociopolitical context of each subject. If framing is shifted in this way, it further pushes the argument of why we should be providing formerly incarcerated persons with the necessary resources toward new potentialities, rather than surveilling them more intensely. Unfortunately, this approach would not only lack political will due to discourses of moral panics and xenophobia but also likely become reconfigured into state practices of surveillance and pathology in order to administer, manage, and control bodies.
As an alternative to reframing the assessment model, the criterion of “risk” could be redefined to include other forms of societal “risk.” In the current models of risk assessment, the focus is on violent offenses. But, what if the criterion shifted to include the risk of the societal economic impact of the offense? That is to say, what if the criterion were redefined and operationalized based on the amount of economic cost an offense is estimated to have on society? It is estimated that the prevalence and cost of white-collar crimes far supersede those of substance use, violent offences, or other non-white-collar crimes. For instance, an estimated $895 billion dollars was lost in 2015 due to fraud. White-collar crimes have a substantial impact on political economy and, as a result, likely the conditions for non-white-collar crimes as well. 104 These are also crimes that are far more prevalent among white businessmen and politicians; thus, the racializations of the algorithms would likely be reconfigured. It is also because of the demographic makeup of these offenses that this approach would not garner much political will. More importantly, it still does not address the oppressive practices of surveilling, managing and controlling marginalized bodies.
Greater mechanisms for auditing administrative data are needed. As each algorithm is being deployed on the decision about any individual, it should be the individual’s constitutional right to see their data and be able to make “objections” or inquiry into any particular aspect of their data. The risk assessments should also be discussed with each individual so that they are informed about how their fate has been predicted. The audit of administrative data should also include open access for community organizations to conduct their own evaluations based on their community’s data. Although administrative data audits are necessary practices for fairness and equity, these practices are also insufficient. Beyond the continued concerns of racialized algorithmic prediction, there remains the economic and legal resources that are necessary to address or redress errors in personal administrative data. These errors include offenses that should have been expunged from an individual’s record, dated personal information, or incorrectly entered information. It is for these reasons that administrative data auditing is both necessary and insufficient for addressing concerns of equity and fairness in predictive policing.
As another practice of transparency, there have been increasing calls for transparency in practices of algorithmic governance. For instance, in August of 2017, a bill was introduced in New York City that would require the city to make public the computer instructions that are employed in government decision-making. 105 This is a policy measure that attempts to create more transparency in the protocological processes of algorithmic governance, a promising direction for enabling greater democratic possibilities. As Galloway cogently argues, it is the protocols of code that are the modulating mechanisms of societies of control. 106 The political discourse on transparency, however, makes at least two problematic assumptions. The first assumption rests on a technologically literate public that is presumed to be able to read and deconstruct the code. The second assumption in this political discourse is that all algorithms are readable and that there are no algorithmic black boxes. Machine learning algorithms such as random forest and neural nets are prime examples of black box algorithms. Once these algorithms are trained on a set of data the probabilistic decision processes of the algorithms are indiscernible, even to the programmer. Thus, efforts for transparency with, for instance, the random forest algorithms of this case study, would be for naught, keeping intact the questionable practices of predictive policing.
It turns out that predictive policing and racial profiling may have the contradictory result of increasing the crime rate rather than decreasing the incidence of crime. As Bernard Harcourt argues in Against Prediction, the panoptic focus on minority groups and communities via predictive policing and racial profiling may result in an increase in the crime rate. He argues that while the profiled groups rate of offending will decrease it will lead to an increased rate of offending in the non-profiled group, which, as the demographic majority in society, will ultimately lead to an overall increase in the rate of offending. Thus, he argues for the use of statistical randomization rather than prediction, whereby everyone will have an equal chance of being surveilled. 107 Although seemingly democratic, we have seen this purportedly implemented with the US Transportation Security Administration at airports, which has been found to still enact practices of racial profiling. Moreover, it is not clear how randomization would work in the context of risk assessments for criminal sentencing or probationary hearings.
As another function of predictive analytics in the context of policing, researchers have proposed and designed data-driven approaches that turn the predictive gaze toward the behaviors of policing. Researchers from the University of Chicago’s Center for Data Science & Public Policy have developed machine learning models that predict which police officers are most likely to be at risk of an adverse event. 108 They are particularly interested in predicting adverse events between the police and the public, such as deadly shootings or racial profiling. Although these sorts of algorithmic practices could have the virtue of both identifying and disciplining some of the policing behaviors that produce sociopolitical relations in the criminal justice system, they also necessitate the institutional force of disciplining. If these predictions were made public and conducted by an independent entity, then this would enable a dimension of Harney and Moten’s non-institutional life. As they argue, “We owe it to each other to falsify the institution, to make politics incorrect, to give the lie to our own determination.” 109 Enabling non-criminal justice entities to estimate these predictions would create conditions for communities to falsify “just” institutional practices of policing. While the level of transparency in the latter is highly unlikely, the former is also fraught with institutional conflicts of interests. Thus, the force of law that is needed in order to reshape the behaviors of policing will be questionable.
While each of the alternative possibilities offer something of equitable utility, they all are limited in their ability to fully redress the problem of perpetuated sociopolitical relations. With the data and the algorithms inheriting the history of the sociopolitical constitution of the human, we argue that the category of the human needs to be reconstituted. A reconstitution of the human would entail a praxis of inquiry that interrogates the sociopolitical code that becomes anchored in the ontogenic flesh. 110 It is only via such a deconstructive process of inquiry that the hegemony of the human and, as such, data and algorithms can be reconfigured toward equitable possibilities. Yet, the reconstitution of the human remains an impossible possibility. Nevertheless, there are alternative possibilities that might work with the racializing assemblages of algorithmic reasoning in order to mitigate the reproducing and reconfiguring of structural relations of difference. This requires an onto-epistemological shift toward algorithms and an understanding of how they performatively intra-act with the world. Only by working with the racializing assemblages of algorithmic reasoning will the necessary calculation enable the provisional conditions of possibility for incalculable justice.
References
Alexander, Michelle. The new Jim Crow: Mass incarceration in the age of colorblindness. The New Press, 2012.
Angwin, Julia, Jeff Larson, Surya Mattu, and Lawrence Kirchner. “Machine Bias.” ProPublica (May 23, 2016). Accessed on October 25, 2016. https://www.propublica.org/article/machine-bias-risk-assessments-in-criminal-sentencing
Babcock, Stephen. “Baltimore’s bail algorithm may not be working as intended.” Technical.ly Baltimore (December 12, 2016). Accessed February 28, 2017. http://technical.ly/baltimore/2016/12/12/bail-decisions-algorithm-citylab/
Barad, Karen. Meeting the Universe Halfway: Quantum Physics and the Entanglement of Matter and Meaning. Durham, N.C.: Duke University Press.
Barnes, Geoffrey C., Lindsay Ahlman, Charlotte Gill, Lawrence W. Sherman, Ellen Kurtz, and Robert Malvestuto. “Low-intensity community supervision for low-risk offenders: a randomized, controlled trial.” Journal of Experimental Criminology 6 (2010): 159-189.
Barnes, Geoffrey C., and Jordan M. Hyatt. Classifying adult probationers by forecasting future offending. Washington, D.C.: National Institute of Justice, 2012. Accessed January 1, 2017. https://www.ncjrs.gov/pdffiles1/nij/grants/238082.pdf
Beck, Allen J. “Prisoners in 2000.” Washington, DC: U.S. Department of Justice, Bureau of Justice Program (2001). Accessed February 28, 2017.
Berk, Richard. “The Role of Race in Forecasts of Violent Crime.” Race and Social Problems 1 (2009): 231-242.
Berk, Richard, Lawrence Sherman, Geoffrey Barnes, Ellen Kurtz, and Lindsay Ahlman. “Forecasting Murder within a Population of Probationers and Parolees: A High Stakes Application of Statistical Learning.” Journal of the Royal Statistical Society 172 (January 2009): 191-211.
Berk, Richard. “Algorithmic criminology.” Security Informatics 2.5 (2013).
Berk, Richard A., Susan B. Sorenson, and Geoffrey Barnes. “Forecasting Domestic Violence: A Machine Learning Approach to Help Inform Arraignment Decisions.” Journal of Empirical Legal Studies 13.1 (2016): 94-115.
Bonilla-Silva, Eduardo. “Rethinking Racism: Toward a Structural Interpretation.” American Sociological Review 62.3 (June 1997): 465-480.
Breiman, Leo. “Random forests.” Machine learning 45.1 (2001): 5-32.
Bureau of Justice Statistics. “Estimated number of inmates held in local jails or under the jurisdiction of state or federal prisons and incarceration rate, 1980-2014.” Bureau of Justice Statistics (July 19, 2016). Accessed March 1, 2017. http://www.bjs.gov/content/keystatistics/excel/Correctional_population_rates_by%20_status_1980-2014.xlsx.
Burgess, Ernest W. “Factors determining success or failure on parole.” The workings of the indeterminate sentence law and the parole system in Illinois (1928): 221-234.
Caesar, Chris. “State explores letting numbers determine risk of defendants jumping bail or re-offending.” Metro (July 14, 2016). Accessed February 28, 2016. http://www.metro.us/boston/state-explores-letting-numbers-determine-risk-of-defendants-jumping-bail-or-re-offending/zsJpgm—GHsJd6WO4RM3
Carton, Samuel, Jennifer Helsby, Kenneth Joseph, Ayesha Mahmud, Youngsoo Park, Joe Walsh, Crystal Cody, CPT Estella Patterson, Lauren Haynes, and Rayid Ghani. “Identifying police officers at risk of adverse events.” kdd.org (2016). Accessed November 1, 2017, http://www.kdd.org/kdd2016/papers/files/adf0832-cartonAemb.pdf.
Cohen, Lawrence E., Marcus Felson, and Kenneth C. Land. “Property crime rates in the United States: A macrodynamic analysis, 1947-1977; with ex ante forecasts for the mid-1980s.” American Journal of Sociology 86.1 (1980): 90-118.
Comte, Auguste. Introduction to Positive Philosophy, trans. Frederick Ferré. Indianapolis: Hackett, 1988.
De Castro, Leandro Nunes. Fundamentals of natural computing: basic concepts, algorithms, and Applications. CRC Press, 2006.
DiCristina, Bruce. “The quantitative emphasis in criminal justice education.” Journal of Criminal Justice Education 8.2 (1997): 181-199.
Dixon-Román, Ezekiel. “Algo-ritmo: More-than-human performative acts and the racializing assemblages of algorithmic architectures.” Cultural Studies↔Critical Methodologies 16.5 (2016): 482-490.
Dixon-Román, Ezekiel. “Toward A Hauntology on Data: On The Sociopolitical Forces of Data Assemblages.” Research in Education 98.1 (2017): 44-58.
Duhigg, Charles, “How companies learn your secret.” The New York Times (February 16, 2012). Accessed December 11, 2016, http://www.nytimes.com/2012/02/19/magazine/shopping-habits.html.
Dwyer, Jim. “Showing the algorithms behind New York City services.” The New York Times (August 24, 2017). Accessed November 1, 2017, https://www.nytimes.com/2017/08/24/nyregion/showing-the-algorithms-behind-new-york-city-services.html.
Foster, Ian, et al., eds. Big data and social science: a practical guide to methods and tools. CRC Press, 2016.
Foucault, Michel, Graham Burchell, Colin Gordon, and Peter Miller. The Foucault effect: Studies in governmentality. University of Chicago Press, 1991.
Foucault, Michel. Security, territory, population: lectures at the Collège de France, 1977-78. Springer, 2007.
Foucault, Michel. The birth of biopolitics: lectures at the Collège de France, 1978-1979. Springer, 2008.
Foucault, Michel. Discipline and punishment: the birth of the prison, trans. Alan Sheridan. New
York: Vintage, 1977.
Galloway, Alexander. Gaming: Essays on Algorithmic Culture. Minneapolis: University of Minnesota Press, 2006.
Gantz, John, and David Reinsel. “Extracting value from chaos.” IDC iview 1142.2011 (2011):1-12.
Gibbons, Don C. Society, crime, and criminal behavior. Englewood Cliffs, NJ: Prentice-Hall,1982.
Harcourt, Bernard. Against Prediction: Profiling, Policing, And Punishing in An Actuarial Age. Chicago, IL: University of Chicago Press, 2006.
Harney, Stefano, & Fred Moten. The Undercommons: Fugitive Planning & Black Study. New York: Minor Compositions, 2013.
Hays, Constance L. “What Wal-Mart knows about customers’ habits.” The New York Times (November 14, 2004). Accessed December 12, 2016. http://www.nytimes.com/2004/11/14/business/yourmoney/what-walmart-knows-about-customers-habits.html?_r=0
Kitchin, Rob. The data revolution: Big data, open data, data infrastructures and their consequences. Sage, 2014.
Laron, Jeff, Surya Mattu, Lauren Kirchner, and Julia Angwin. “How We Analyzed the COMPAS Recidivism Algorithm.” ProPublica (May 23, 2016). Accessed on October 25, 2016. https://www.propublica.org/article/how-we-analyzed-the-compas-recidivism-algorithm
LoBianco, Tom. “Report: Nixon’s war on drugs targeted black people.” CNN (March 24, 2016).Accessed February 28, 2017. http://www.cnn.com/2016/03/23/politics/john-ehrlichman-richard-nixon-drug-war-blacks-hippie/.
McCarthy, John. “What is artificial intelligence.” Stanford University Formal Reasoning Group, November 12, 2007. Accessed February 1, 2017. http://www-formal. stanford.edu/jmc/whatisai. html, 38.
McKee, Guian A. “Lyndon B. Johnson and the war on poverty: Introduction to the digital edition.” The University of Virginia Press, 2010. Accessed December 12, 2016. http://presidentialrecordings.rotunda.upress.virginia. edu/essays.
Michell, Joel. Measurement in psychology: A critical history of a methodological concept.Cambridge University Press, 1999.
Mills, Steve, Steve Lucas, Leo Irakliotis, Michael Rappa, Teresa Carlson, and Bill Perlowitz. “Demystifying big data: a practical guide to transforming the business of government.” Washington, D.C.: TechAmerican Foundation, 2012.
Moynihan, D. P. “The Negro family: The case for national action (a report for the US Department of Labor, Office of Policy Planning and Research).” Washington, DC: Government Printing Office, 1965.
Northpointe. “COMPAS: The Most Scientifically Advanced Risk and Needs Assessments.” Accessed February 28, 2017. http://www.northpointeinc.com/risk-needs-assessment
Parisi, Luciana. Contagious architecture: Computation, aesthetics and space. Cambridge, MA: The MIT Press, 2013.
Perry, Walter L., Brian McInnis, Carter C. Price, Susan C. Smith, and John S. Hollywood. “Predictive policing: The role of crime forecasting in law enforcement operations.” Rand Corporation, 2013.
Porter, Theodore M. Trust in numbers: The pursuit of objectivity in science and public life. Princeton University Press, 1996.
PredPol. “About PredPol.” Accessed February 28, 2017. http://www.predpol.com/about/
Quinlan, John R. “Simplifying Decision Trees.” International journal of Man-Machine Studies 27.3 (1987): 221-234.
Schmidt, Peter, and Ann Witte. Predicting recidivism using survival models. New York: Springer-Verlag, 2012.
Statistical Atlas. “Philadelphia – Income.” Accessed February 28, 2017. http://statisticalatlas.com/place/Pennsylvania/Philadelphia/Household-Income#data-map/neighborhood
Statistical Atlas. “Philadelphia – Race and Ethnicity.” Accessed February 28, 2017. http://statisticalatlas.com/place/Pennsylvania/Philadelphia/Race-and-Ethnicity#data-map/neighborhood
TechAmerica Foundation. “Demystifying big data: a practical guide to transforming the business of government.” Washington (DC): TechAmerica Foundation (2012).
Travis, Jeremy, Bruce Western, and Steve Redburn. The growth of incarceration in the United States: Exploring causes and consequences. Washington, D.C.: The National Academies Press, 2014.
Uchida, Craig D. “Predictive Policing in Los Angeles: Planning and Development.” Justice & Security Strategies (December 2009).
United States Census Bureau. (2002). U.S. Summary: 2000 (C2KPROF/00-US) [Census 2000 profile]. Accessed February 28, 2017. https://www.census.gov/prod/2002pubs/c2kprof00-us.pdf.
United States Congress. (1968). “Omnibus crime control and safe streets (pp. 1-48).” Accessed on February 28, 2017. http://transition.fcc.gov/Bureaus/OSEC/library/legislative_histories/1615.pdf.
University at Albany, Hindelang Criminal Justice Research Center. Table 6.28.2012. Sourcebook of Criminal Justice Statistics. Accessed February 13, 2017. http://www.albany.edu/sourcebook/pdf/t6282012.pdf.
Weheliye, Alexander G. Habeas Viscus: Racializing Assemblages, Biopolitics, and Black Feminist Theories of the Human. Durham, NC: Duke University Press, 2014.
Weiss, Rick, and Lisa-Joy Zgorski. “Obama administration unveils ‘big data’ initiative: Announces $200 million in new R&D investments.” Office of Science and Technology Policy, Executive Office of the President (March 12, 2012). Accessed February 28, 2017. https://obamawhitehouse.archives.gov/the-press-office/2015/11/19/release-obama-administration-unveils-big-data-initiative-announces-200
Woolley, John T., and Gerhard Peters. “Lyndon B. Johnson: Special message to the Congress on crime and law enforcement.” American Presidency Project. Accessed December 11, 2016. http://www.presidency.ucsb.edu/ws/?pid=27478.
WSRP. “New study confirms the prevalence and cost of white collar crime.” WSRP (May 31, 2016). Accessed November 1, 2017, https://www.wsrp.com/new-study-confirms-prevalence-cost-white-collar-crime/.
Wynter, S. “Human Being as Noun? Or Being Human as Praxis? Towards the Autopoetic Turn/Overturn: A Manifesto.” 2007. Available at: https://www.scribd.com/document/329082323/Human-Being-as-Noun-Or-Being-Human-as-Praxis-Towards-the-Autopoetic-Turn-Overturn-A-Manifesto#from_embed (last accessed May 19, 2017)
Zikopoulos, Paul, and Chris Eaton. Understanding big data: Analytics for enterprise class hadoop and streaming data. McGraw-Hill Osborne Media, 2011.
Notes
- Richard Berk, “Algorithmic criminology,” Security Informatics 2.5 (2013). ↩
- Craig D. Uchida, “Predictive Policing in Los Angeles: Planning and Development,” Justice & Security Strategies (December 2009). ↩
- Richard A. Berk, Susan B. Sorenson, and Geoffrey Barnes, “Forecasting Domestic Violence: A Machine Learning Approach to Help Inform Arraignment Decisions,” Journal of Empirical Legal Studies 13.1 (2016), 96. ↩
- Ezekiel Dixon-Román, “Algo-ritmo: More-than-human performative acts and the racializing assemblages of algorithmic architectures,” Cultural Studies↔Critical Methodologies 16.5 (2016). ↩
- Luciana Parisi, Contagious Architecture: Computation, aesthetics and space, 2013. ↩
- Alex Wehelive, Habeas Viscus: Racializing Assemblages, Biopolitics, and Black Feminist Theories of the Human, 2014. ↩
- Berk, Sorenson, and Barnes, “Forecasting Domestic Violence.” ↩
- Charles Duhigg, “How companies learn your secret,” The New York Times (February 16, 2012). ↩
- Constance L. Hays, “What Wal-Mart knows about customers’ habits,” The New York Times (November 14, 2004). ↩
- Rick Weiss and Lisa-Joy Zgorski, “Obama administration unveils ‘big data’ initiative: Announces $200 million in new R&D investments,” Office of Science and Technology Policy, Executive Office of the President (March 12, 2012). ↩
- E.g., Ernest W. Burgess, “Factors determining success or failure on parole,” The workings of the indeterminate sentence law and the parole system in Illinois (1928): 221-234; Don C. Gibbons, Society, crime, and criminal behavior, 1982. ↩
- E.g., Cohen, Lawrence E., Marcus Felson, and Kenneth C. Land, “Property crime rates in the United States: A macrodynamic analysis, 1947-1977; with ex ante forecasts for the mid-1980s,” American Journal of Sociology 86.1 (1980): 90-118; Peter Schmidt and Ann Witte, Predicting recidivism using survival models, 2012. ↩
- E.g., Bruce DiCristina, “The quantitative emphasis in criminal justice education,” Journal of Criminal Justice Education 8.2 (1997). ↩
- Auguste Comte, Introduction to Positive Philosophy, trans. Frederick Ferré, 1988. ↩
- Joel Michell, Measurement in psychology: A critical history of a methodological concept, 1999. ↩
- Theodore Porter, Trust in Numbers, 1996. ↩
- Ibid., 8. ↩
- Ibid. ↩
- Ibid., 45. ↩
- Ibid., 77. ↩
- Michel Foucault, Discipline and Punish, 1977, 170, 183. ↩
- Foucault, Security, Territory, Population: lectures at the Collège de France, 2007, 26. ↩
- Foucault et al, The Foucault effect: Studies in governmentality, 1991; Foucault, Security, Territory, Population ↩
- Guian A. McKee, “Lyndon B. Johnson and the war on poverty: Introduction to the digital Edition,” 2010. ↩
- Michelle Alexander, The New Jim Crow, 2010. ↩
- Daniel Moynihan, “The Negro Family: The case for national action,” 1965. ↩
- Alexander, The New Jim Crow; United States Congress, “Omnibus crime control and safe streets (pp. 1-48),”1968. ↩
- For a brief discussion of President Johnsons’ thoughts on the correlates of crime, see John T. Woolley and Gerhard Peters, “Lyndon B. Johnson: Special message to the Congress on crime and law enforcement,” American Presidency Project. ↩
- Jeremy Travis, Bruce Western, and Steve Redburn, The growth of incarceration in the United States: Exploring causes and consequences, 2014, 110. ↩
- Ibid., 111. ↩
- Ibid. ↩
- Bureau of Justice Statistics, “Estimated number of inmates held in local jails or under the jurisdiction of state or federal prisons and incarceration rate, 1980-2014” (2016). ↩
- Travis, Western, and Redburn, The growth of incarceration in the United States. ↩
- United States Census Bureau, U.S. Summary: 2000 (2002); Allen J. Beck, “Prisoners in 2000,” 2001. ↩
- United States Census Bureau, US Summary: 2000; Allen J. Beck, “Prisoners in 2000,” 2001. ↩
- Tom LoBianco, “Report: Nixon’s war on drugs targeted black people,” CNN (March 24, 2016). ↩
- Walter L. Perry et al, “Predictive policing: The role of crime forecasting in law enforcement operations,” 2013; Uchida, “Predictive Policing in Los Angeles.” ↩
- Perry et al., “Predictive Policing.” ↩
- Ibid. ↩
- John McCarthy, “What is artificial intelligence,” 2007. ↩
- Ian Foster et al, Big Data and Social Science: A Practical Guide to Methods and Tools, 2016, 148. ↩
- Ian Foster et al, Big Data and Social Science: A Practical Guide to Methods and Tools, 2016, 148. ↩
- Leandro Nunes De Castro, Fundamentals of natural computing: basic concepts, algorithms, and Applications, 2006. ↩
- Ian Foster et al, Big Data and Social Science: A Practical Guide to Methods and Tools, 2016, 148. ↩
- Ian Foster et al, Big Data and Social Science: A Practical Guide to Methods and Tools, 2016, 148. ↩
- e.g., Perry et al., “Predictive Policing.” ↩
- In Massachusetts: Chris Caesar, “State explores letting numbers determine risk of defendants jumping bail or re-offending,” Metro (July 14, 2016); in Baltimore: Stephen Babcock, “Baltimore’s bail algorithm may not be working as intended,” Technical.ly Baltimore (December 12, 2016). ↩
- Philadelphia provides the most salient example of this particular application; we discuss this case in-depth below. ↩
- PredPol, “About PredPol,” retrieved from http://www.predpol.com/about/. ↩
- Ibid., “How PredPol Works,” retrieved from http://www.predpol.com/how-predpol-works/ ↩
- Northpointe, “COMPAS: The Most Scientifically Advanced Risk and Needs Assessments,” accessed February 28, 2017, http://www.northpointeinc.com/risk-needs-assessment. ↩
- Dixon-Román, “Algo-ritmo.” ↩
- Rob Kitchin, The data revolution: Big data, open data, data infrastructures and their Consequences, 2014. ↩
- Dixon-Román, “Toward A Hauntology on Data.” ↩
- Parisi, Contagious architecture. ↩
- Karen Barad, Meeting the Universe Halfway: Quantum Physics and the Entanglement of Matter and Meaning, 2007. ↩
- Wehelive, Habeas Viscus, 2014. ↩
- Ibid., 3. ↩
- Quoted in Weheliye, Habeas Viscus, 39. ↩
- Geoffrey C. Barnes and Jordan M. Hyatt, Classifying Adult Probationers by Forecasting Future Offending: Final Technical Report (2012). ↩
- Geoffrey C. Barnes et al., “Low-intensity community supervision for low-risk offenders: A randomized, controlled trial,” Journal of Experimental Criminology 6 (2010). ↩
- Richard Berk et al., “Forecasting Murder within a Population of Probationers and Parolees: A High-Stakes Application of Statistical Learning,” Journal of the Royal Statistical Society 172 (2009); Richard Berk, “The Role of Race in Forecasts of Violent Crime,” Race and Social Problems 1 (2009). ↩
- For methodology, see Jeff Larson, et al., “How We Analyzed the COMPAS Recidivism Algorithm,” ProPublica (May 23, 2016); for full article, see Julia Angwin, et al., “Machine Bias,” ProPublica (May 23, 2016). ↩
- Berk et al., “Forecasting Murder,” 192. ↩
- Barnes and Hyatt, Classifying Adult Probationers by Forecasting Future Offending, 3. ↩
- John R. Quinlan, “Simplifying Decision Trees”, International journal of Man-Machine Studies 27.3 (1987): 221-234. ↩
- Barnes and Hyatt, Classifying Adult Probationers by Forecasting Future Offending. ↩
- Foster et al., Big Data and Social Science: A Practical Guide to Methods and Tools. ↩
- Barnes and Hyatt, Classifying Adult Probationers by Forecasting Future Offending; Leo Breiman, “Random forests,” Machine learning 45.1 (2001): 5-32. ↩
- Barnes and Hyatt, Classifying Adult Probationers by Forecasting Future Offending, 5. ↩
- Berk, “The Role of Race,” 232. ↩
- e.g. Berk, “The Role of Race.” ↩
- Roughly 85 percent of the data sample used was reported to be African American; see Berk, “Forecasting Murder,” 233. ↩
- See Table 1, Barnes et al., “Low-intensity community supervision for low-risk offenders,” 172-3. ↩
- Ibid., 173. ↩
- By contrast, a commentary on the skewness of the age variable among the study sample as compared to the overall APPD caseload appears in the study’s conclusion on page 185 as a possible limitation. ↩
- Barnes and Hyatt, Classifying Adult Probationers by Forecasting Future Offending, 60. ↩
- Berk et al., “Forecasting Murder,” 207. ↩
- Ibid., 207. ↩
- Barnes and Hyatt, Classifying Adult Probationers by Forecasting Future Offending, 38. Emphasis added. ↩
- Berk, “Forecasting Murder,” 238. ↩
- Eduardo Bonilla-Silva, “Rethinking Racism: Toward a Structural Interpretation,” American Sociological Review 62.3 (June 1997). ↩
- The casual way in which the race of study participants is presented in Barnes et al. (2012) as just another baseline demographic feature to report in Table 1 (p. 172-3) is a good example of this treatment of race in the texts. ↩
- See Barnes and Hyatt, Classifying Adult Probationers by Forecasting Future Offending, 6. ↩
- Berk et al., “Forecasting Murder,” 206. ↩
- Barnes and Hyatt, Classifying Adult Probationers by Forecasting Future Offending, 8. ↩
- ↩
- See Barnes and Hyatt, Classifying Adult Probationers by Forecasting Future Offending, “Statement of Hypotheses,” 15; Barnes et al., “Low-intensity community supervision for low-risk offenders,” 183. ↩
- Richard Berk, “Algorithmic Criminology,” Security Informatics 2 (2013). ↩
- Barnes et al., “Low-intensity community supervision for low-risk offenders,” 185. ↩
- Berk et al., “Forecasting Murder,” 208. ↩
- Ibid., 206. ↩
- Barnes et al., “Low-intensity community supervision for low-risk offenders,” 168. ↩
- Census data retrieved from Statistical Atlas: Racial data retrieved from http://statisticalatlas.com/place/Pennsylvania/Philadelphia/Race-and-Ethnicity#data-map/neighborhood and income data retrieved from http://statisticalatlas.com/place/Pennsylvania/Philadelphia/Household-Income#data-map/neighborhood. ↩
- Barnes and Hyatt, Classifying Adult Probationers by Forecasting Future Offending, 24. ↩
- For an overview of these data samples, see Barnes and Hyatt, Classifying Adult Probationers by Forecasting Future Offending, 7-8 (construction sample) and 10 (validation sample). ↩
- Ibid., 10. ↩
- Angwin et al., “Machine Bias.” ↩
- e.g., Barnes et al., “Low-intensity community supervision for low-risk offenders.” ↩
- Berk, “The Role of Race.” ↩
- Barnes and Hyatt, Classifying Adult Probationers by Forecasting Future Offending, 28-29. ↩
- Berk, “The Role of Race,” 232. ↩
- See discussions of Models A, B, and C in Barnes and Hyatt report. ↩
- WSRP, “New study confirms the prevalence and cost of white collar crime,” WSRP (May 31, 2016). ↩
- Jim Dwyer, “Showing the algorithms behind New York City services,” The New York Times (August 24, 2017). ↩
- Alexander Galloway, Gaming: Essays on Algorithmic Culture, 2006. ↩
- Bernard Harcourt, Against Prediction: Profiling, Policing, And Punishing in An Actuarial Age, 2006. ↩
- Samuel Carton et al, “Identifying police officers at risk of adverse events,” 2016. ↩
- Stefano Harney and Fred Moten, The Undercommons: Fugitive Planning & Black Study, 2013, 20. ↩
- Silvia Wynter, “Human Being as Noun? Or Being Human as Praxis? Towards the Autopoetic Turn/Overturn: A Manifesto,” 2007. ↩